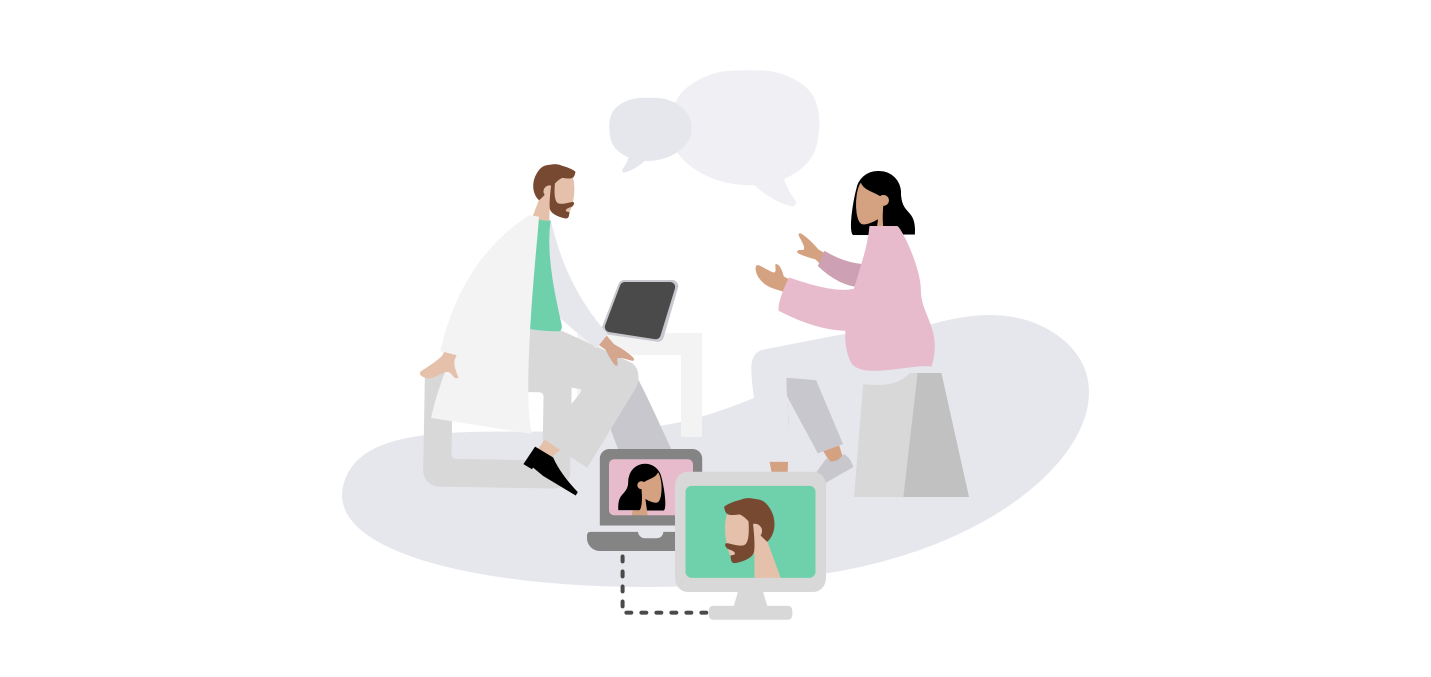
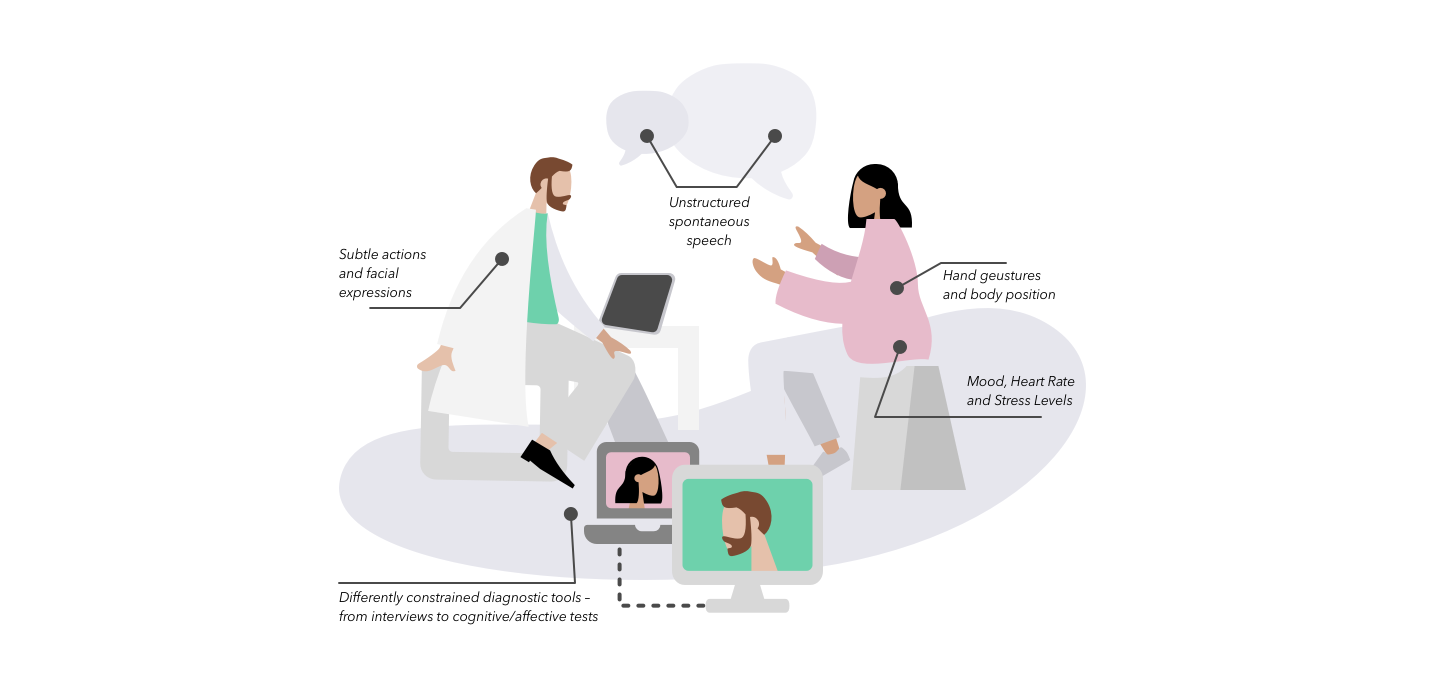
The vision of MEPHESTO is to help break the the scientific ground for the next generation of precision Psychiatry through AI-based social interaction analysis.
MEPHESTO seeks to bring digital neuroscience to ecologically valid real-life care situations specifically in clinical social interactions by introducing multi-modal sensors in a minimally invasive manor. Social interactions like the conversation between patient and doctor are traditionally a clinician’s most important source of information especially in psychiatry. By moving beyond isolated read outs from artificial laboratory settings and subjective patient self-reports or even clinician-based assessments, this project aims to give clinicians quantitative measures to better understand and address psychiatry needs.
For context, a digital phenotype is similar to a phenotype derived from biological blood samples. But rather it is the footprint of a pathology on a digital data stream such as audio, video, physiology activity. To build these digital phenotypes multiple scientific domains must efficiently collaborate: computer vision, speech analysis, behavioural science, medicine just to name a few.
At the very core of MEPHESTO is the development of scientifically sound and clinically valid phenotypes for psychiatric disorders based on multimodal inputs from clinical social interactions. For this purpose, a multi-site, multinational, cross-sectional as well as longitudinal study is being conducted that collects data from video recordings, conversations, but also traditional Bio-signals. This project has built a covid-considerate clinical data collection setup comprised of 2 kinetic cameras to record audio and video as well as wearable bio-sensors on the wrist to collect hear rate and skin perspiration.
Short Term Objectives| Establishing an ambitious multidisciplinary research core group between INRIA and DFKI. As well as laying the foundation for generating future value from scientific excellence. Pushing the interdisciplinary research on novel medical AI applications by leveraging INRIA’s (STARS) expertise in computer vision and DFKI’s knowledge on speech and dialogue analysis with the goal of clinical interaction analysis.
Long Term Objectives| Building novel scientific expertise at the interface of computer vision, computational linguistics, psychology, sociology and medicine (neurology & psychiatry). From this scientific ecosystem, generate applied and innovation projects (e.g. via EIT Health, other funding schemes) and economic value (e.g. start-up creation & industrial projects).
Positive Symptoms in Schizophrenia | Objective measurement of positive symptoms in Schizophrenia through automatic speech analysis
Supporting Differential Diagnosis | Supporting differential Diagnosis for major depressive episode eitology through combined analysis of video, audio and physiology data
Quantify Therapeutic Alliance | Which multimodal measure are indicative for interaction quality levels between clinicians and patients?
Relapse Prediction | Prediction of symptoms progress & treatment response; definition of digital phenotypes (over time)
König, A. et al. (2022). “Multimodal Phenotyping of Psychiatric Disorders From Social Interaction: Protocol of a Clinical Multicenter Prospective Study.” Personalized Medicine in Psychiatry
Müller, P. et al. (2022). “MultiMediate’22: Backchannel Detection and Agreement Estimation in Group Interactions.” In Proceedings of the 30th ACM International Conference on Multimediate.
Balazia, M. et al. (2022). “Bodily Behaviors in Social Interaction: Novel Annotations and State-of-the-Art Evaluation.” In proceedings of the 30th ACM International Conference on Multimedia.
Abdou, A. et al. (2022). “Gaze-enhanced Crossmodal Embeddings for Emotion Recognition.” In Proceedings of the ACM on Human-Computer Interaction 6.ETRA (2022): 1-18.
Ettore, E. et al. (2022). “Digital phenotyping for differential diagnosis of Major Depressive Episode: A Literature Review.” (Preprint).
Lindsay, H. et al. (2022). “Generating Synthetic Clinical Speech Data Through Simulated ASR Deletion Error.” In Proceedings of the RaPID-4 at the 13th LREC.
Lindsay, H. et al. (2021). “Multilingual Learning for Mild Cognitive Impairment Screening from a Clinical Speech Task.” International Conference Recent Advances in Natural Language Processing.
Lindsay, H. et al. (2021). “Language Impairment in Alzheimer’s Disease—Robust and Explainable Evidence for AD-Related Deterioration of Spontaneous Speech Through Multilingual Machine Learning“. Frontiers in Aging Neuroscience.
Müller, P. et al. (2021). “MultiMediate: Multi-modal Group Behaviour Analysis for Artificial Mediation.” In ACM International Conference on Multimedia.